Optimizing Business Operations with Data Labeling in Machine Learning
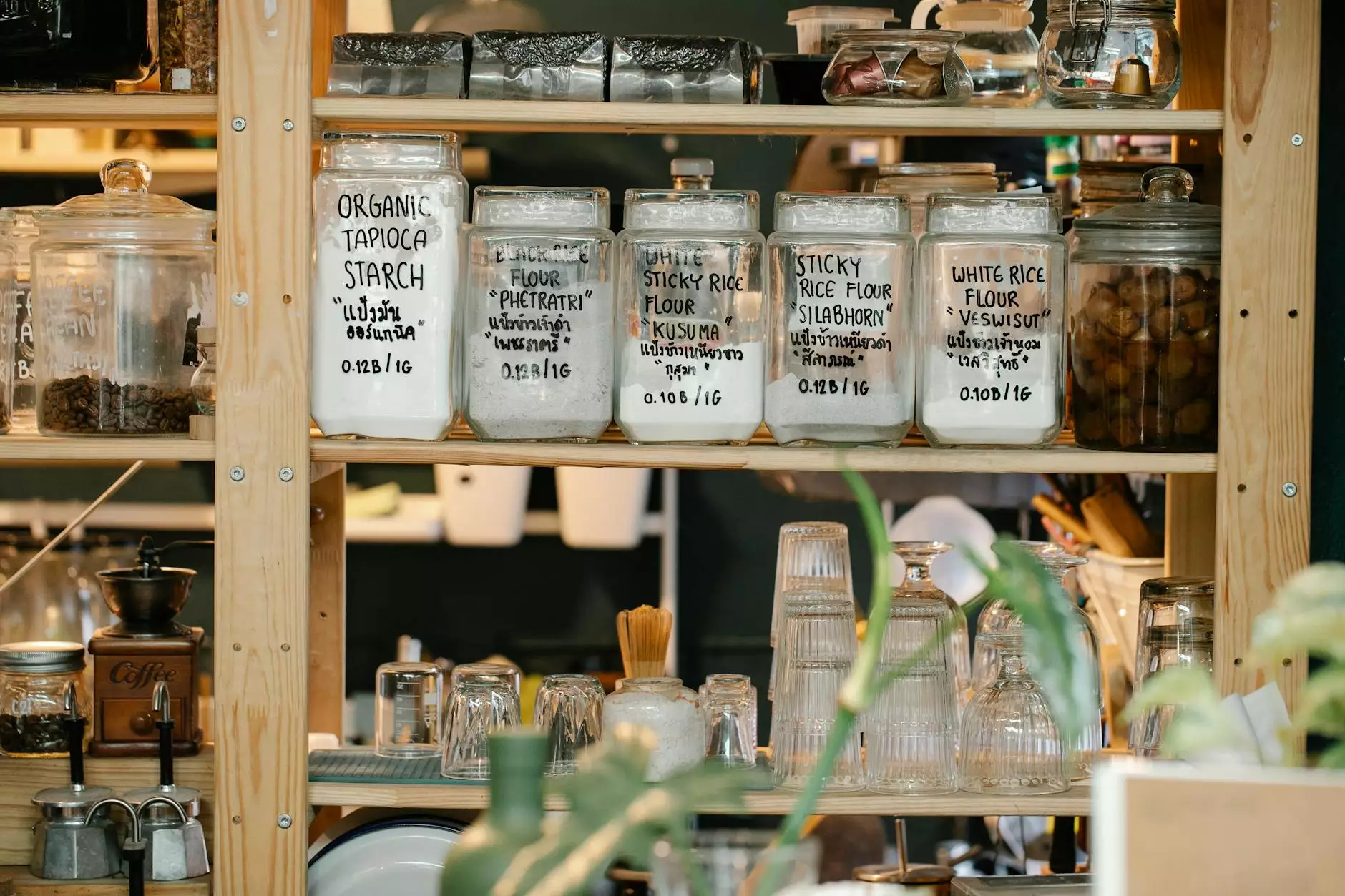
In the rapidly evolving landscape of technology, businesses are continually seeking innovative methods to enhance their operations and serve their customers more efficiently. One such innovation is the integration of data labeling within the field of machine learning. This article will delve into the significance of data labeling, especially for businesses in the sectors of home services, keys, and locksmiths, and how it can propel operational excellence.
Understanding Data Labeling in Machine Learning
Data labeling is a critical process in machine learning that involves identifying and tagging data to make it understandable for algorithms. In simpler terms, data labeling transforms raw data into a format that machines can learn from. This might include labeling images, annotating text, or categorizing audio. The effectiveness of machine learning models largely hinges on the quality and accuracy of the labeled data used in their training.
The Role of Data Labeling in Businesses
For businesses, especially those in sectors like home services, keys, and locksmiths, data labeling serves multiple purposes:
- Enhancing Customer Experience: By leveraging machine learning models trained on accurately labeled datasets, businesses can predict customer needs and tailor their services accordingly.
- Improving Operational Efficiency: Automation processes can be designed to streamline workflows by utilizing machine learning algorithms that learn from well-labeled data.
- Reducing Errors: Accurate data labeling minimizes the chances of errors in decision-making processes, which can be particularly critical in areas such as security and emergency locksmith services.
How Data Labeling Works
The data labeling process typically follows several structured steps:
1. Data Collection
Businesses begin by gathering relevant data. This could include images of locks, audio recordings of customer inquiries, or service transaction logs.
2. Annotation
In this phase, the collected data is annotated, or labeled, by human workers. This might involve drawing bounding boxes around items in images or transcribing voice notes into text data.
3. Quality Assurance
To ensure the accuracy of the labels, a quality assurance step is crucial. This process may involve multiple reviews of the labels to identify and rectify any discrepancies.
4. Training Machine Learning Models
Once the data is accurately labeled, it can be used to train machine learning models, which will learn to recognize patterns within the data and make predictions based on new inputs.
The Importance of High-Quality Data Labeling
In the realm of data labeling for machine learning, quality is paramount. Poorly labeled data can lead to significant issues:
1. Misleading Outcomes
Machine learning models trained on inaccurate data can produce misleading outcomes, affecting decision-making processes and business strategies.
2. Increased Operational Costs
Incorrect predictions may necessitate remedial actions, resulting in additional labor costs and resource allocation.
3. Damage to Reputation
In industries such as locksmithing, where trust is critical, delivering subpar service due to flawed data can irreparably damage a business's reputation.
Technologies Behind Data Labeling
As we progress further into the digital age, the technologies behind data labeling continue to evolve. Here are some noteworthy innovations:
1. Automated Data Labeling Tools
Recent advancements have led to the development of automated data labeling tools that can significantly accelerate the labeling process. By using AI and pre-trained models, businesses can auto-label data, saving time and resources.
2. Crowdsourcing
Crowdsourcing has emerged as an effective way to label vast amounts of data. Through platforms that connect businesses with freelance annotators, high-quality labeled data can be obtained at scale.
3. Active Learning
This is a machine learning paradigm where the model identifies which data points it is uncertain about and requests labels for those specific examples, optimizing the labeling process.
Benefits of Data Labeling for Home Services, Keys & Locksmiths
For businesses operating in home services, keys, and locksmiths, the benefits of effective data labeling are manifold:
1. Enhanced Predictive Analytics
By analyzing trends and customer preferences, businesses can effectively predict consumer behavior. This allows for proactive service offerings, ensuring that customers receive the help they need when they need it.
2. Improved Service Offerings
Data insights gained from machine learning can guide businesses in optimizing their service offerings, ensuring they address the most critical and demanded services in their locality.
3. Increased Competitive Advantage
Utilizing data-driven insights allows locksmiths and home service providers to stay ahead of their competition, providing tailored services that meet the unique demands of their client base.
Incorporating Data Labeling into Business Strategy
For businesses in the home services and locksmith sectors, integrating data labeling into their operational strategy involves:
1. Identifying Key Data Points
Determine what data is most relevant to your operations. This could involve analyzing customer interaction data, service completion times, or service feedback.
2. Choosing the Right Labeling Tools
Selecting appropriate tools and technologies for data labeling that suit your organizational needs, budget, and scale of data.
3. Continuous Training and Updating
Machine learning models need to be regularly trained with new data to remain relevant. Establishing a routine for updating data labels is crucial for ongoing accuracy.
Conclusion: A Future-Ready Business
In conclusion, data labeling is a fundamental aspect of implementing machine learning solutions that can significantly drive growth and efficiency in the business realm. For companies involved in home services, keys, and locksmiths, embracing this technology can lead not only to operational success but also to enhanced customer satisfaction and loyalty.
The future of business operations lies in leveraging advanced technologies such as data labeling in machine learning. By investing in high-quality data labeling processes and tools, businesses can equip themselves to respond to increasingly complex market demands and position themselves as leaders in their fields.
For more insights on data labeling and how it can transform your business, visit keymakr.com.
data labeling machine learning