Unlocking the Potential of Medical Datasets for Machine Learning
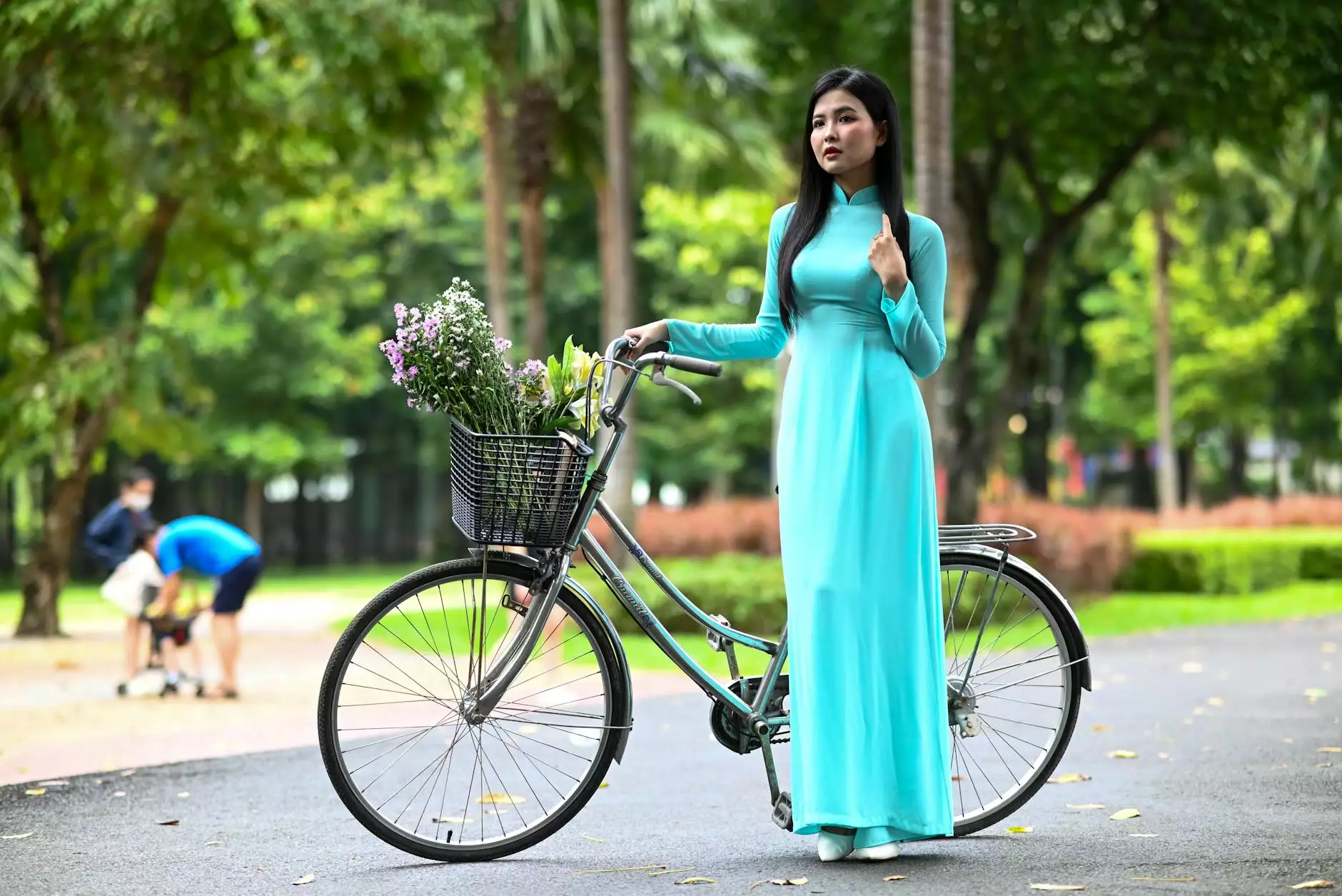
The emergence of machine learning has revolutionized various sectors, and the healthcare industry is no exception. The utilization of medical datasets for machine learning applications has made significant strides in enhancing medical practices, improving patient outcomes, and streamlining operations. In this article, we delve into the invaluable role of medical datasets in machine learning, exploring their applications, benefits, and the ethical considerations essential for their effective use.
Understanding Medical Datasets
At its core, a medical dataset for machine learning refers to a collection of health-related data that can be used to train machine learning algorithms. These datasets include various types of information such as:
- Patient Records: Comprehensive details of patients' medical histories, treatments, and outcomes.
- Clinical Trials Data: Results from clinical experiments focused on testing new drugs or procedures.
- Diagnostic Data: Information gathered from medical imaging, pathology reports, and lab tests.
- Genomic Data: Data derived from sequencing genomes, providing insights into genetic factors affecting health.
Each category of data contributes uniquely to the machine learning ecosystem, enabling researchers and practitioners to develop insightful models that aid in diagnosis, prediction, and personalized treatment plans.
Applications of Medical Datasets in Machine Learning
The applications of medical datasets for machine learning are vast and varied. Here are some key areas where this technology is making a profound impact:
1. Predictive Analytics
One of the most significant advantages of using medical datasets is the ability to perform predictive analytics. Machine learning models can analyze historical patient data to predict health outcomes, such as:
- Risk of diseases like diabetes or heart disease.
- Potential hospital readmissions based on past patient records.
- Response to particular medications or treatment plans.
By leveraging these insights, healthcare providers can intervene proactively, ultimately improving patient care and reducing costs.
2. Diagnostic Assistance
Machine learning algorithms trained on extensive medical datasets can significantly enhance diagnostic accuracy. For instance, algorithms can be developed to:
- Analyze medical images, such as X-rays and MRIs, with remarkable precision.
- Identify patterns in lab results that may indicate the presence of a disease.
- Support doctors in determining the best course of treatment based on comparable case studies.
This not only aids healthcare professionals but also helps in reducing the diagnostic errors associated with human oversight.
3. Personalized Medicine
The shift towards personalized medicine is another groundbreaking application of medical datasets for machine learning. By analyzing genomic data along with patient records, healthcare providers can tailor treatments specifically to individual patients, considering factors such as:
- Genetic predispositions to certain diseases.
- Past responses to treatments within similar patient demographics.
- Unique biostatistical factors influencing health outcomes.
This approach leads to more effective treatment plans and improved patient compliance and satisfaction.
4. Operational Efficiency
Machine learning can also enhance the operational efficiency of healthcare facilities. By analyzing administrative data, facilities can optimize:
- Staffing levels based on patient volume predictions.
- Supply chain management for medical resources.
- Scheduling systems to reduce patient waiting times.
Such improvements not only reduce costs but also enhance the overall patient experience.
Ethical Considerations in Using Medical Datasets
While the benefits of utilizing medical datasets for machine learning are immense, there are ethical considerations that must be addressed to ensure responsible use. These include:
1. Patient Privacy
Maintaining patient confidentiality is paramount. Medical datasets often contain sensitive personal information that must be protected through:
- Data anonymization techniques to remove personally identifiable information.
- Strict access controls to limit who can view and analyze the data.
- Compliance with regulations such as HIPAA to safeguard patient rights.
2. Data Bias
Machine learning algorithms are inherently dependent on the data they are trained on. If the medical dataset is biased, it may lead to skewed outcomes. To mitigate these risks, it is essential to:
- Utilize diverse datasets that represent various demographics.
- Regularly audit algorithms for fairness and accuracy in predictions.
- Engage ethicists and data scientists in the process of dataset selection and model training.
3. Informed Consent
Informed consent is critical when using patient data for research and analysis. Patients should:
- Be made aware of how their data will be used.
- Have the opportunity to opt-out of data sharing initiatives at any time.
- Receive information on the potential benefits and risks associated with data usage.
Future Trends in Machine Learning and Medical Datasets
The future of machine learning in the healthcare sector is poised for remarkable developments thanks to advancements in technology and the continuous evolution of medical datasets. Here are several trends to watch for:
1. Integration of IoT Devices
With the rise of the Internet of Things (IoT), medical devices are becoming increasingly interconnected. Future datasets will include real-time data from wearable devices, enhancing predictive capabilities and allowing for more immediate interventions.
2. Enhanced Data Interoperability
As healthcare providers adopt various electronic health record (EHR) systems, the push for standardized data formats will grow. This will facilitate the sharing and integration of datasets across platforms, leading to richer datasets for machine learning applications.
3. AI-Driven Diagnostics
Artificial intelligence (AI) will play a crucial role in automating diagnostic processes. Machine learning algorithms will evolve to not only assist but also autonomously diagnose certain conditions based on comprehensive datasets.
4. Predictive Health Monitoring
Advancements in machine learning will enable sophisticated models that predict health issues before they manifest symptomatically. Such predictive health monitoring could transform preventive care and wellness strategies.
Conclusion
The use of medical datasets for machine learning is revolutionizing the healthcare landscape, presenting both opportunities and challenges. By harnessing the power of these datasets, healthcare providers can significantly improve patient outcomes, optimize operations, and contribute to a more efficient healthcare system. However, the path forward requires a commitment to ethical practices, data privacy, and continuous improvement to mitigate risks. As technology progresses, the potential of medical datasets is boundless, and we stand at the forefront of a transformative era in healthcare.
For more insights and updates on Home Services, Keys & Locksmiths, stay connected with us at keymakr.com. Together, we can unlock the future of healthcare and technology!